Workshops & Seminare
Die folgenden Kurse sind für Wissenschaftlerinnen und Wissenschaftler gedacht, die ein solides Verständnis der geeigneten statistischen Methoden aufbauen wollen, um aus den eigenen Daten in ihrem jeweiligen Arbeitsgebiet neue Erkenntnisse zu gewinnen. Dies bietet eine fundierte Grundlage für eine gute statistische Praxis und die Generierung von datengetriebener Evidenz durch Forschung.

Grundlagen der Biostatistik
Dieser Kurs vermittelt jungen Forschern in den Naturwissenschaften ein grundlegendes Verständnis der Statistik.
- Deskriptive Statistik & Graphiken
- Prizip des statistischen Testens
- Statistical tests & interpretation
- Evaluation von Fold-Change Daten
- Korrelation und Regression
- Studiendesign und gängige Stolpersteine

Gute statistische Praxis
Hilfestellung für Forscher beim Verstehen der häufigsten Fallstricke und Missverständnisse bei der Durchführung statistischer Analysen und der Kommunikation von Ergebnissen.
- Identifizieren uninformativer oder irreführender Diagramme
- Hierarchische Datenstrukturen
- P-werte und ihre Einschränkungen
- Statistische Significanz vs. biologische Relevance
- Adjustierung für multiples Testen

Fortgeschrittene Biostatistik
Dieser Kurs befasst sich mit fortgeschrittenen biostatistischen Fragestellungen einschließlich einiger theoretischer Hintergründe und praktischer Anwendungen.
- ANOVA & Gruppentests
- Regressionsmodelle
- Supervidierte Methoden (Klassifikation & Vorhersage)
- Unsupervidierte Anstätze (Clustering, PCA, ...)

Einführung in statistische Prgrammierung mit R
Eine sanfte Einführung in die Funktionsweise von R und Rstudio zur Datenanalyse.
- Objekte, Funktionen & Hilfe
- Datenimport
- Deskriptive Statistik
- Erste graphische Darstellungen
- Variablentransformationen
- Eigene Funktionen schreiben
- Erste statistische Analysen
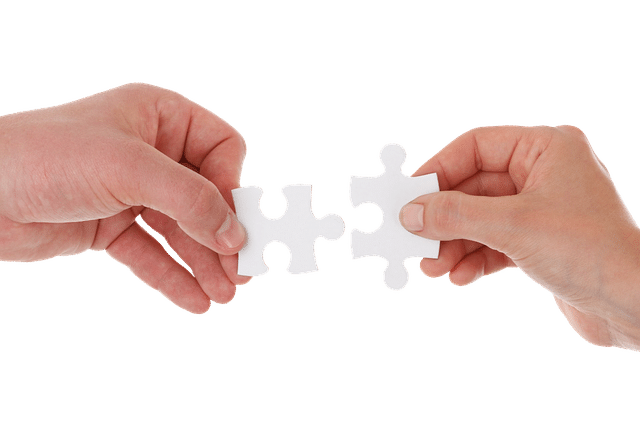

Identifikation von Subgruppen mittles Omics-Daten
Ein Überblick über unsupervidierte Methoden zur Identifizierung von Untergruppen in Omics-Daten mit Hilfe von Bioconductor und der Programmiersprache R.
- Unsupervidiertes Lernen
- Clustering Techniken
- Dimensionsreduktion
- Visualisierung & Interpretation
- Validität und Stabilität
Icons licensed under CC BY 4.0. © Laura Reen
Genau das ansprechen, was Ihre Teilnehmer brauchen...
Das Spektrum der behandelten Themen, Inhalte und die Dauer des Workshops können so angepasst werden, dass sie genau auf die Bedürfnisse Ihrer Teilnehmer zugeschnitten sind. Setzen Sie sich mit mir in Verbindung und lassen Sie mich wissen, welche Themen für Ihre Gruppe am relevantesten sind.
Was die Teilnehmer sagen
Super zufrieden mit dem Kurs! Themen, von denen ich dachte, dass sie sehr schwierig sein würden, wurden sehr gut erklärt.
Enthusiastischer Referent! Es hat immer Spaß gemacht, zuzuhören, und ich habe viel gelernt.
Dank der Energie des Trainers, der bisher interessanteste Statistikkurs!
Great course, perfect lecturer, easy to understand. Now I know what the p-value is.
Häufig gestellte Fragen
Die Kursgrößen variieren je nach Format und Kursinhalt.
Für Statistikkurse mit im Seminarformat, wie z.B. Grundlagen der Biostatistik und gute statistische Praxis, empfiehlt sich eine Teilnehmerzahl zwischen 10 und 50 Personen. Diese Gruppengröße ermutigt die Teilnehmer oftmals miteinander zu interagieren und sich an Diskussionen zu beteiligen.
Für Seminare mit praktischen Programmierübungen (z.B. Einführung in R, Analyse von Omics-Daten und Identifizierung von Subgruppen) sind Gruppengrößen von 8 bis 20 Personen vorzuziehen. Dadurch wird sichergestellt, dass individuelle Programmierfragen schnell angesprochen und gelöst werden können.
The statistics courses, such as Basic Principles of Biostatistics and Good statistical practice, are intended for young life-science researchers and do not require any particular statistical knowledge. High school level mathematics suffices, as these seminars intend to establish an understanding of basic statistical aspects that researchers are confronted with during their own work and in scientific publications.
Ebenso ist der Workshop Einführung in R für absolute Anfänger gedacht und erfordert keine Vorkenntnisse in der Programmierung. Die Teilnehmer sollten ihren eigenen Laptop für die Übung mitbringen.
The seminars involving programming sessions with Bioconductor (e.g. Analysis of Omics data, and Subgroup Identification) require a basic understanding of statistical concepts and some prior experience with R programming. These courses are not useful for participants with no knowledge of R. Participants should bring their own laptop for the practical session.
Bei allen meinen Kurstypen bin ich bestrebt, ansprechende und lohnende Lernerfahrungen zu vermitteln. Ich ermutige die Studierenden zur Diskussion und Interaktion durch den Einsatz von Umfrage-Software, die von bisherigen Teilnehmern begeistert aufgenommen wurde. Ich finde es auch wichtig, eine einladende Atmosphäre zu schaffen und genügend Zeit für Interaktion und Fragen einzuplanen.
Wenn ich einen vollen Seminarraum vor mir sehe, ist mir bewusst, dass sich jeder Teilnehmer aus extra Zeit seinem vollen Terminkalender genommen hat, um diesen Kurs zu besuchen. Daher ist es mein Ziel, das Seminar zu einer bereichernden Erfahrung zu machen, indem ich auf die Bedürfnisse der Teilnehmer eingehe und dafür sorge, dass sie die richtigen statistischen Werkzeuge erhalten, die sie benötigen, um erfolgreiche Wissenschaftler zu werden.
Das Honorar variiert je nach Kursinhalt, Format und Länge. Setzen Sie sich mit mir in Verbindung, um Ihren speziellen Fall zu besprechen, und ich werde Ihnen gerne einen Kostenvoranschlag unterbreiten.
Ja, alle Kurse können je nach den Präferenzen der Teilnehmer entweder auf Deutsch oder auf Englisch gehalten werden.
Ich kann einigermaßen flexibel sein und mich nach Ihrem Veranstaltungskalender richten. Lassen Sie uns kurz darüber sprechen, welche Termine Sie im Auge haben, und ich werde sehen, was ich tun kann.
Die Seminare finden in der Regel in Ihrem Institut bzw. Ihren Räumlichkeiten statt, sowohl um die Organisation zu erleichtern als auch um Kosten zu sparen - ich bin jedoch offen für andere Optionen, die Sie vielleicht besser geeignet finden.
Ich habe meinen Sitz in der Nähe von Heidelberg und kann überall in Deutschland und der Europäischen Union Kurse geben. Orte wie Frankfurt, Darmstadt, Mainz, Wiesbaden und Mannheim sind innerhalb einer Stunde leicht zu erreichen, so dass ich an diesen und ähnlichen Orten keinen Hotelübernachtung benötige.
Ausgedehntere Reisen können gern in Betracht gezogen werden, erfordert jedoch eine angemessene Vorankündigung und Unterstützung auf Ihrer Seite.
Ein normaler Konferenzraum ist völlig ausreichend: ein Projektor, gute Sicht und Akustik, WiFi.
Für Seminare mit praktischen Programmiersitzungen (z.B. Einführung in R, Analyse von Omics-Daten und Identifizierung von Subgruppen) werden ausreichend Steckdosen für Laptops der Teilnehmer benötigt.